Markets Media spoke with Ashok Reddy, CEO of KX about the criticality of merging temporal intelligence with ultra-fast data processing and analytics to transform capital markets.
What key market factors are driving financial institutions to adopt AI solutions?
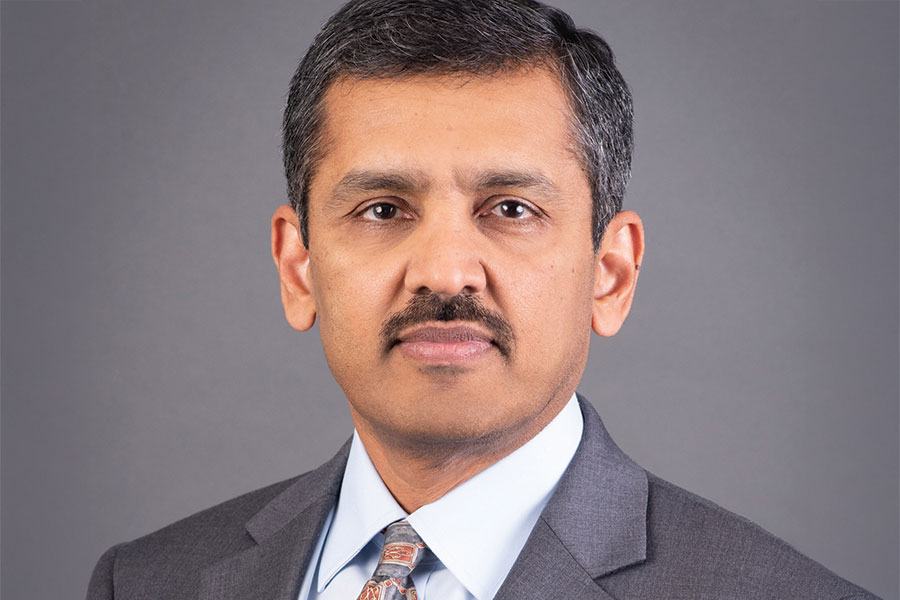
Machine learning algorithms and discriminative AI have long been mission-critical in capital markets. These firms use specialised technology, like we offer at KX, to process massive datasets, find patterns and make predictions with speed and accuracy.
Today, financial institutions are increasingly adopting AI solutions due to a convergence of market forces that demand greater speed, precision, and efficiency in a world where milliseconds can mean millions. As market velocity accelerates, and scalability and adaptability become critical qualities that move AI from experimentation into production, firms must break through what we call the ‘AI sound barrier,’ where traditional data infrastructure creates drag that prevents organisations from fully harnessing their time-sensitive data. At the same time, increasing regulatory scrutiny is pushing financial institutions to adopt AI models that are both auditable and compliant.
AI can transform financial operations by augmenting research, generating and debugging code for financial models, streamlining documentation and enhancing pattern recognition and contextual processing.
Customers increasingly value personalised services and community engagement from their financial institutions and by combining the creativity of generative AI with the precision of analytical AI, banks can deliver real-time, highly accurate insights that transform customer experiences by allowing then to refine products, optimise onboarding, and adapt services to evolving needs – ensuring relevance, precision, and personalisation at every critical moment.
Why is the “when” of data just as important as the “what” in AI-driven trading decisions, and how does this temporal dimension create competitive advantages?
In capital markets, success hinges on precise timing – understanding when a signal emerges can be the difference between a profitable trade and a missed opportunity. AI models that incorporate temporal intelligence enable firms to learn, predict and act in real time.
Temporal AI goes beyond traditional time-series AI by handling asynchronous events (not just evenly spaced time steps), causal reasoning (how past events drive future outcomes) and adaptive learning (models that evolve over time). The temporal dimension is the key to real-time intelligence, autonomous decision-making, and next-gen AI applications that will give companies the ability to act on the dynamics of time-driven data faster than anyone else.
How are the demands of temporal-aware AI reshaping technology infrastructure requirements for trading firms?
The demands of temporal-aware AI require cloud-native architectures, vector databases and high-performance data engines. High-performance time-series databases are becoming essential as they enable seamless handling of sequential market data. Firms are investing in low-latency processing engines to support real-time trading decisions while ensuring their AI models provide explainability and regulatory transparency.
Effective AI depends on accurate and complete data, as well as the latency and throughput to generate rapid insights. It’s about completeness, timeliness and efficiency – or, to put it another way, having all the data, exactly when you need it, delivered most effectively.
Beyond raw computing power, what foundational capabilities must firms establish to effectively implement AI systems that position companies to beat their competitors time and again?
Financial firms are increasingly seeking AI systems that combine structured numerical computation with unstructured data processing in real time. This reflects a shift toward more versatile, context-aware architectures, or, hybrid AI.
True numerical computation is critical, as LLMs (such as ChatGPT) rely on statistical pattern recognition rather than performing real mathematical calculations. AI models must also be capable of processing sequential time-series data to avoid misleading predictions caused by disordered inputs.
Additionally, regulatory explainability is paramount – financial institutions cannot afford to rely on opaque, black-box models that offer no visibility into their decision-making processes. Scalable, low-latency processing capabilities further ensure that AI-driven trading strategies remain competitive in fast-moving markets. KX’s software addresses these requirements by providing firms with the infrastructure necessary to make informed, high-speed financial decisions while maintaining compliance and transparency.
What are the biggest data-related challenges in applying temporal AI to real-time trading environments?
Applying temporal AI to real-time trading environments presents several challenges, mostly related to data accuracy, computational integrity, and latency constraints. AI models require precise timestamps and consistent data streams to generate reliable market insights. However, as research by Bradford Levy demonstrates, LLMs often fail at basic numerical reasoning, meaning they cannot be relied upon for financial decision-making. Additionally, commercial LLMs exhibit significant look-ahead bias, meaning their seemingly strong performance may be due to implicit knowledge of future outcomes rather than true predictive ability.
Compounding the issue, these models struggle with time-series forecasting. Since LLMs fail to retain and understand the sequential nature of data, their ability to generate reliable financial predictions is severely constrained. Financial strategies often depend on precise timing, but GenAI models fundamentally lack the temporal awareness needed to interpret long-term dependencies. To combat this, purpose-built platforms are emerging to enable more reliable, explainable outputs in regulatory environments.
KX’s high-performance analytics engine is designed to ingest, analyse, and act on time-sensitive data at unmatched speed, ensuring that trading firms maintain a real-time competitive advantage. Unlike traditional solutions that treat time as just another data point, our approach understands how data changes over time, providing crucial context for predictions and decisions.
How can organisations better structure their data architecture to support the precise timing needs of modern AI trading algorithms?
To support the precise timing needs of modern AI trading algorithms, organisations must structure their data architecture with a focus on real-time efficiency. Time-series databases provide the foundation for accurate market predictions by allowing AI models to process data in a strictly sequential manner. This requires moving beyond surface-level data accuracy to ensure that the data truly reflects the temporal nuances needed for effective decision-making. Optimising data pipelines for ultra-low latency ensures that AI-driven trading systems can react instantaneously to new information.
A hybrid AI approach – where analytical AI handles rigorous computation, and GenAI assists with research and code automation – ensures that firms benefit from both precision and adaptability. Built-in auditability and explainability are becoming foundational requirements as regulatory expectations increase in both scope and sophistication. Compliance requirements necessitate AI models that provide clear, auditable reasoning for their trading decisions. Utilising a platform that is purpose-built for these types of challenges allows organisations to manage complex financial data with the precision and speed required for success.
Looking at successful implementations, how are firms that effectively merge temporal intelligence with ultra-fast processing gaining measurable performance advantages over competitors?
Firms that effectively merge temporal intelligence with ultra-fast processing gain measurable performance advantages over their competitors in the following ways:
- Enhanced Predictive Capabilities: By understanding temporal patterns, AI can make more accurate predictions about future events.
- Improved Anomaly Detection: Temporal context allows AI to identify anomalies that occur over time
- More Sophisticated Decision-Making: AI can make more nuanced and context-aware decisions by considering the temporal relationships between events.
- Personalisation: By understanding a user’s past history, and how that history changes over time, AI can provide very highly personalised real time responses such as dynamic portfolio adjustments or real-time onboarding optimisation.
Superior trade execution timing enables them to act milliseconds ahead of the market, leading to increased profitability. Additionally, firms leveraging real-time AI are better equipped to mitigate risks by responding to market shifts as they happen rather than after the fact. The ability to provide clear, explainable decision-making also reduces regulatory risks, ensuring compliance with evolving financial regulations.
kx.com