The advice of pre-trade models should be taken with a hefty grain of salt, according to speakers at this year’s TradeTech – particularly during times of volatility, where prior trade performances will not necessarily repeat themselves.
“Pre-trade models are crucial, but they should be an indication rather than absolute answers,” said Sam Vaughan-Jones, senior equity dealer at Royal London Asset Management.
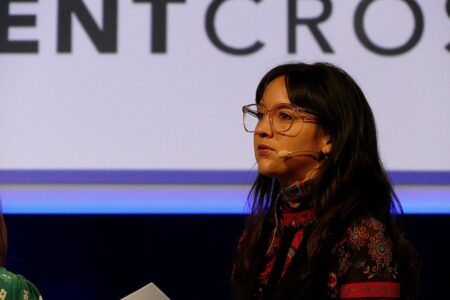
In volatile conditions, the performances of historical trades are not reliable indicators of future outcomes. While pre-trade models can be tweaked, Danielle Fregeau, global equity trader at Impax Asset Management, stated that she pivots away from pre-trade analytics in volatile conditions. “It’s hard to get them adapted quickly,” she noted. “To lean on those models is a comfort, but you have to take them with a grain of salt. They could lead you down a trickier path.”
“The models go out the window when volatility is high,” Vaughan-Jones agreed. While their insights are not entirely ignored, traders’ situation-specific decisions are more highly valued.
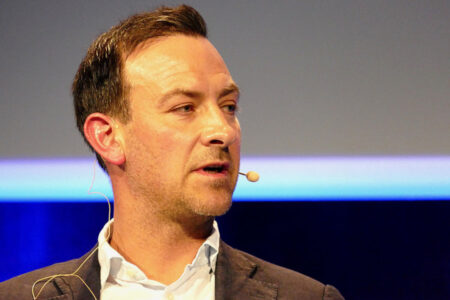
Beyond unexpected market conditions, pre-trade models can also be destabilised by a lack of, or inconsistencies in, the data they run on.
“We cannot get real-time fills from one of the brokers we are currently using,” Fregeau said, something “critical” on the high-touch desk. “That kills our post-trade processes and means that we have to be very manual. Then, we cannot feed the results into a pre-trade model.”
Similarly hampering efficiency are inconsistent tagging processes across firms.
“There are differences across the buy side as to how the portfolio manager can convey instructions to traders, and then to the counterparty. We’re miles away from having a standardised approach that would facilitate harmonised transaction cost analysis,” noted Benjamin Mahe, head of high-touch trading at AXA Investment Managers.
In turn, this makes it difficult to create more comprehensive pre-trade models.
“You have to work with the data you have,” Fregeau said. “It’s hard to replicate a trade on a given day – you have to think about expectations for the trade, then look back and reassess.”
While AI and large language models (LLMs) are an inescapable talking point across the industry, Vaughan-Jones assured: “we wouldn’t use them in our pre-trade models.” LLMs may be used as a guide, but during volatility trust in these programmes declines.
“We’re not using AI in pre or post trade,” he continued. “AI is a reason that markets have become more efficient, but it has also increased volatility.”
Fregeau was more optimistic towards LLM use, stating that Impax uses the technology in third-party cost models to establish expected costs and measure how executions perform
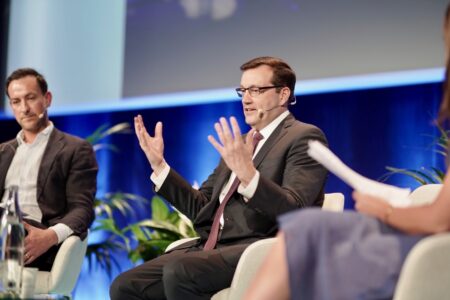
against that.
From a vendor perspective, Kevin O’Connor, global head of analytics at Virtu, agreed that pre-trade models should be seen as decision-support tools rather than predictive models and called for a change of mindset around the technology.
Panelists did not comment on the particular vendors they used for pre-trade models.
Whether vendor or client, the industry agrees that pre-trade models are advisory rather than prescriptive. While technology and data analytics are developing at pace, they are far from being able to predict the future.