investors face the challenge of managing overwhelming data volumes while finding ways to gain a competitive advantage.
By Tony McManus, Global Head of Bloomberg’s Enterprise Data Business
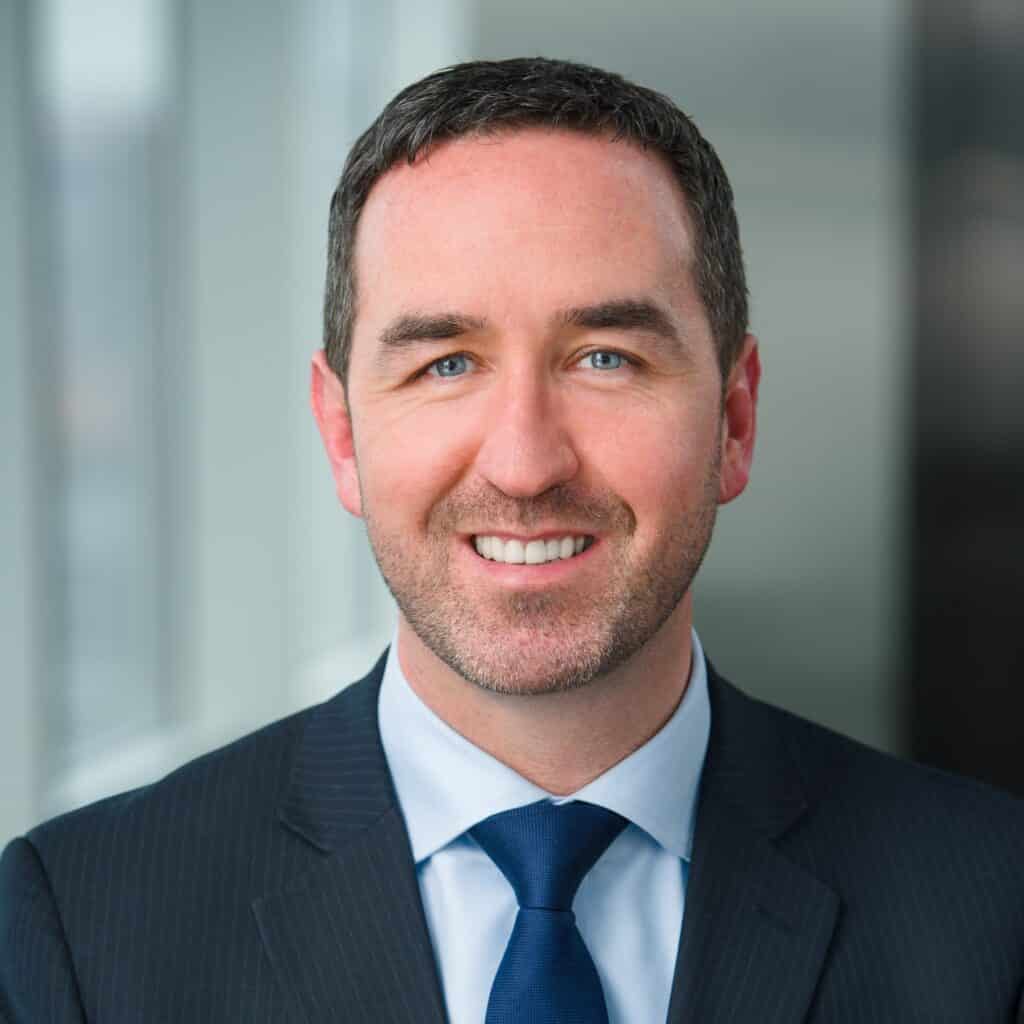
Today’s institutional investors are steadily becoming much more data driven and quantitative in their investment management strategies. The combination of infinite computing power, data-friendly programming languages, machine learning tools, advances in AI and easy access to financial analytics have collectively reduced barriers to entry and unlocked an abundance of new data sources. Even more traditional fundamental investors are implementing quantitative techniques — a practice the industry has dubbed “quantamental” investing.
For example, fundamental investors demand even longer histories now to ensure their signals are adaptive to different market regimes or sudden shocks, like those we’ve seen in the last couple of years. They are also looking beyond narrow company-level data into big picture macro indicators, as well as signals from the options and credit markets, to understand how companies they hold will be impacted.
Many segments of the financial services industry also face intense competition and cost pressure and are looking to recent advances in AI technology to enable more efficient, cost-effective services. To benefit from the use of AI, investors need a large volume of machine readable, high-quality data with rich history and that data needs to be easily accessible.
But in a world where data is more ubiquitous and accessible than ever before, investors face the dual challenge of managing the overwhelming volume and finding ways to derive a material competitive advantage. Markets are non-stationary, with changing drivers — exemplified by major concerns and influences today such as inflation, fears of recession and deterioration of credit conditions. Asset class and factor returns are also cyclical and time-varying, and past effective signals by nature are subject to performance decay. Each of these factors contributes to investors’ perpetual need for fresher, more granular data. And while each investor’s approach and priorities are unique, the ongoing hunt for differentiated, value-adding data presents similar challenges across the industry.
Navigating a vast sea of complex data
The cost and burden of acquiring, mapping, cleansing and normalizing the staggering amount of data within investors’ reach has become a herculean task. Moreover, simply acquiring data and applying standard data science techniques won’t deliver the results investors are looking for. Buy-side firms are continuously monitoring for more granular data, distinctive signals within datasets, and/or unique combinations of data to yield new perspectives that translate to returns. Here are three challenges that data-driven investors must conquer to unlock value in today’s financial markets:
1. Authentic point-in-time data is essential, but scarce.
As many quants will know, “point-in-time” data is critical for ensuring accurate backtesting, reducing the potential for overfitting and avoiding various biases such as look-ahead bias and survivorship bias. However, many data providers do not offer true, historical point-in-time data.
Consider a scenario where a company releases an earnings statement and then issues a revision the following week. True historical point-in-time data would reflect both statements and account for their impact on the market. However, many providers would not store these two different earnings reports as separate records, instead only providing the corrected record. Firms relying upon this data would not be able to accurately recreate original conditions, potentially resulting in look-ahead bias in models and overstating expected returns.
2. Sourcing and organizing information from multiple data providers is onerous, complex and expensive.
In order to glean valuable new insights that the market hasn’t already uncovered, investors need to combine multiple datasets to achieve a precise, comprehensive view of company performance. Each dataset offers its own nuances, and the process of collating data from different providers and packaging it in a form that is useful to analysts is complex. Given that this requires dealing with different identifiers and structures, it’s also a heavy lift on the data engineering side. In fact, it is commonly accepted that firms spend dramatically more time, effort and money acquiring, ingesting, cleansing, normalizing, cataloging and organizing data than they do on analyzing it.
3. To gain a competitive edge, investors need ever-deeper levels of granularity.
The global macroeconomic environment is becoming increasingly complex. The diverse economic responses to inflation, as well as specific regional pressures (for example, energy prices in Europe), demand that investors understand the detailed macroeconomic picture (including productivity, employment, spending and inflation data) at the local level.
Individual companies are also becoming more complex as they expand and diversify their products and services. Investors need a level of data granularity that reflects this complexity and enables analysis for prediction of future potential performance. For instance, Disney’s holdings include theme parks, cruise ships and a streaming service. Financial analysts need segment-level data about each of these completely different businesses to evaluate the company’s overall performance.
Finally, a granular micro-view of a single company can provide a game-changing competitive edge. Symphony Health, which helps companies gain deep insight into the pharmaceutical market, has historical data on prescriptions. A financial analyst who aggregated that data could tell how certain drugs are performing, which would indicate what medicines doctors are prescribing. From there, the quant could discern insights about the likely performance of certain pharmaceutical companies that other firms may not have considered.
The trend toward ever more data-driven investing is perhaps best exemplified by the deluge of data that has accompanied the integration of ESG factors into portfolios. In fact, data has become so plentiful that the age-old problem of scarcity has been replaced by the opposite conundrum: when access to information is limitless, harmonizing, normalizing and gleaning insights from data represents a formidable challenge. In this environment, especially with advances in AI technology, the investors who figure out how best to parse that data using an optimal mix of tools, strategy and partnerships will be the ones who carve out a competitive advant